AI Acceleration: Keeping Supply Chains in Sync with Fast-Paced Engineering BOMs
- Stefan Schmeisser
- Nov 7, 2023
- 2 min read
Updated: Nov 7, 2023
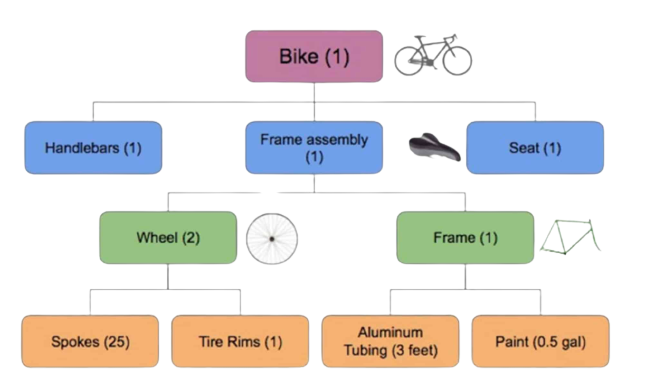
In today's example, let us dive into a scenario that is all too familiar: An engineering organization is tasked with developing a new product.
This happens all the time. Product management identifies a new market opportunity and provides requirements to engineering. Meanwhile, Supply Chain is tasked with sourcing the components of this new product.
Engineering and Supply Chain align and identify those critical components that must be sourced and somewhat cross their fingers on the rest of the BOM.
At his stage in the product development cycle, the BOM is like a living, breathing entity—it's ever-changing. Components are added and removed with dizzying frequency as the engineering team embraces a "fail fast" philosophy. Meanwhile, supply chain and material managers are chasing cost targets for components that may or may not be on the final BOM.
What you need in this fast-paced development frenzy is equally swift technology to give the supply chain folks a fighting chance to dance in step with engineering.
Modern rapid development requires modern technology to enable a supply chain organization to "keep up" with engineering
Our software deploys several AI models that get down to business by sifting through mechanical drawings, extracting the essential bits of data, which can then be arranged into "live" business intelligence dashboards. This kind of intelligence is pure gold—it allows the supply chain to keep pace!
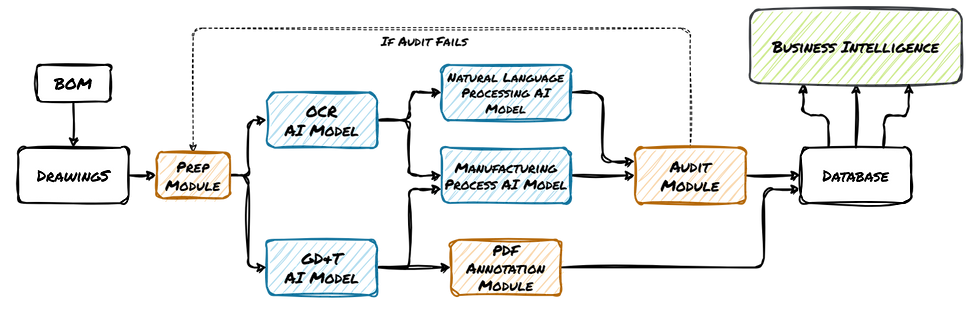
Low-Hanging Fruit: A dashboard providing a clear picture of your new product BOM by sorting components via Raw Material and Size
Just by teasing out dimensions and raw material specifications from each component in the BOM, we can illuminate an understanding of the manufacturing requirements for the entire product.

Craving deeper insights into your new product? Extract even more requirements!
Sure, Raw Material and size play a major role when selecting suppliers, but you can dig a little deeper into the manufacturing needs for each piece of the BOM.
In the following example, we are using SourceOptima's extracted dimensions, tolerance requirements, and identified callouts on each component to make some assumptions. Assumptions that each organization can, of course, establish for themselves using their own rules:
Dimension extraction sizes up each part as small, medium, or large.
Tolerance details sort the parts into "Difficult" for tight tolerances and "Not difficult" for more generous ones.
GD&T callouts are spotted and tagged. To keep things simple, let's say a component with 10 or fewer callouts is "Simple," while anything over 11 callouts is "complex."

Additionally, we are extracting process and finish requirements. You can see that these two requirements sometimes get mixed up. As we continue to train our AI models, we can differentiate between the "process" and "finish" with greater success. However, any supply chain manager will gladly overlook it to appreciate this level of granularity today, generated with the click of a button!
In conclusion, the future of manufacturing is nimble, responsive, and data-driven—and with solutions like SourceOptima, companies are well-equipped to thrive in this environment. So whether you're looking for the low-hanging fruit or aiming for the stars, it's clear that embracing intelligent technology is the key to keeping up with the ceaseless tempo of modern product innovation.
Comentarios